In this last chapter of our Maintenance Stories campaign, Mohamed Sallak, Ph.D. and Associate Professor/HRD at the Heudiasyc laboratory of the University of Compiègne (FRANCE), proposes a focus on predictive maintenance. Too often confused with condition-based maintenance, it is often misused. How to avoid mistakes and how to perfectly position it in your strategy? Our ITD Research expert explains.
©Photo: Doyle Shin
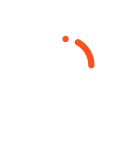
Definition
Predictive maintenance is defined by the norm EN 13306 [1] as condition-based maintenance carried out following a forecast derived from the analysis and evaluation of the significant parameters of the degradation of the item. According to this definition, predictive maintenance typically involves:
- Condition monitoring: activity performed either manually or automatically, intended to measure at predetermined intervals the characteristics and parameters of the physical actual state of an item.
Fault diagnosis: actions taken for fault recognition, fault localization, and identification of causes. - Fault prognosis: actions taken for predicting a future state (of reliability) based on current and historic conditions, or estimating the Remaining Useful Life (RUL) of items.
- Maintenance plans: structured and documented set of tasks that include the activities, procedures, resources, and the time scale required to carry out maintenance.
Essentially, predictive maintenance can be divided into the following three technical basics, based on their data sources:
- The existing sensor-based maintenance technique
- The test-sensor-based maintenance technique
- The test signal-based maintenance technique
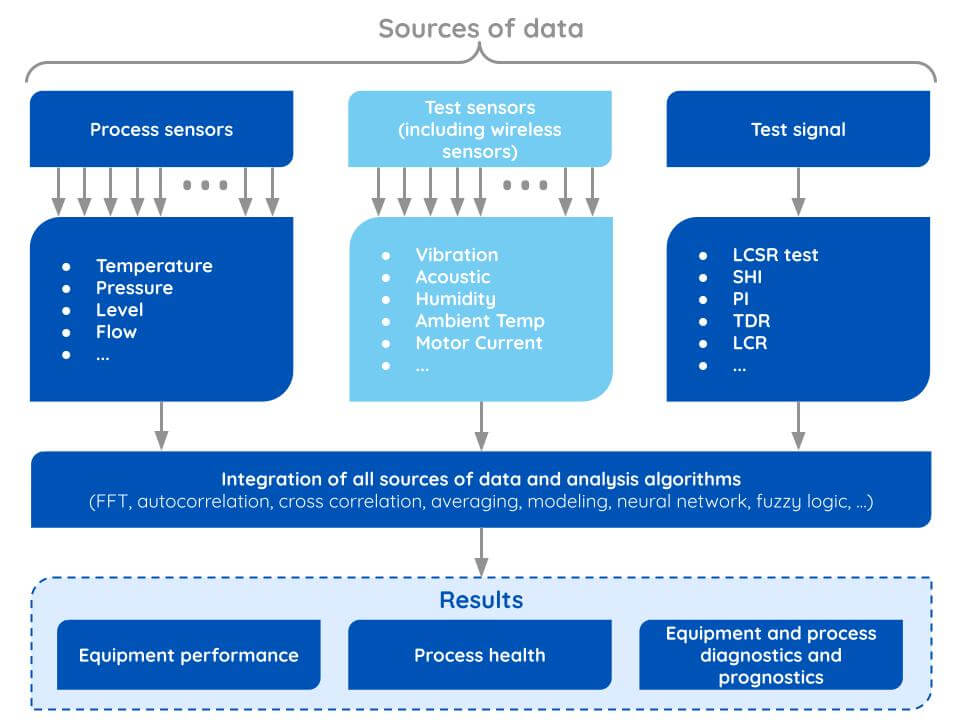
Predictive maintenance vs. condition-based maintenance
Condition-based Maintenance (CBM) defined by the European norm EN13306 as preventive maintenance which includes the assessment of physical conditions, analysis, and the possible ensuing maintenance actions, is typically confused with predictive maintenance.
In fact, even if condition-based maintenance and predictive maintenance share the same overarching goals mainly in reducing equipment downtime and optimizing resources by performing maintenance work only when needed, there is a slight difference between both approaches: while predictive maintenance uses sensor measurements combined with precise algorithmic formulas to predict the precise moment for maintenance action, condition-based maintenance can only provide information when given physical parameters go below a certain threshold, and action needs to be taken in the present moment to avoid equipment failure.
Thus, predictive maintenance is a more advanced form of maintenance than condition-based maintenance.
Types of predictive maintenance
Imperfect maintenance is a new kind of maintenance approach, which has spread in the recent decades, as an alternative to the common classification of maintenance, proposed for example by the EN13306. Predictive maintenance can be perfect or imperfect. A perfect predictive maintenance action restores the system to the “as good as new” state, while imperfect predictive maintenance restores the system to a state between “good-as-new” and “bad-as-old”.
More specifically, considering the main parameters defining the reliability features of the system, we could say that after a perfect maintenance action, a system has the same lifetime distribution and the same failure rate function like a new one. For this reason, generally, the replacement of a system by a new one is a perfect repair.
The imperfect predictive maintenance may accelerate the deterioration process of the system. The implementation of perfect maintenance policies seems quite simple. However, perfect maintenance actions are often expensive. Moreover, imperfect maintenance has grown recently as a popular issue to researchers as well as industrial applications.
A comparison with other types of maintenance
Predictive maintenance allows teams to overcome the drawbacks of corrective maintenance and preventive maintenance by avoiding equipment failure and providing optimal use of the equipment at the same time. While corrective maintenance leads to high risks of equipment failure and maintenance costs, preventive maintenance leads to reduced risks of equipment failure (cf. figure below). However, preventive maintenance may engender high costs of maintenance related to poor maintenance management. Predictive maintenance allows teams to achieve an optimal combination of both maintenance strategies in terms of maintenance cost and failure risk.
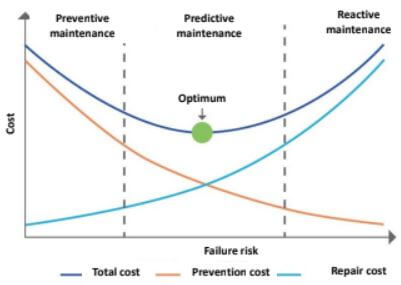
Evaluate remaining useful life with predictive maintenance
Predictive maintenance is generally based on the estimation of the remaining useful life (RUL) of equipment. The RUL is a frequently used measurement to perform failure prognosis. It is one of the key factors in condition-based maintenance, prognosis, and health management. It is defined as the expected length of time the system or component is likely to operate before it fails. The RUL can be expressed as follows:

where E[X] is the function that gives the expected value of a random variable X and f is the density function of failure distribution and S is the duration life span of the equipment.
In other terms, RUL is the expected time remaining for a system to perform its functional capabilities. It can be also defined as the duration from the current time to the end of useful life for a system.
It is obvious that RUL is a decreasing function in time. The RUL is an important functional aspect of an efficient prognosis and health monitoring in predictive maintenance. In fact, the prediction of RUL is necessary to verify if the mission goal can be accomplished on time and is important to help in online decision-making activities such as fault mitigation, mission replanning, etc. Therefore, it is more used in prognosis and health monitoring than other physical metrics such as failure rate or reliability. However, the estimation of RUL is rarely done with complete precision since we are dealing with future predictions. There is always a dispersion associated with the estimated value of RUL and even if we try to minimize this dispersion, there is always a risk that the real value of RUL is out of the interval of RUL dispersion.
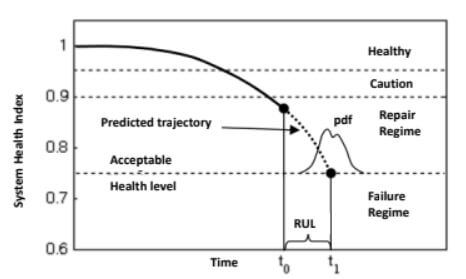
Benefits of ClickOnSite predictive maintenance
ClickOnSite provides the following benefits when used for predictive maintenance:
- ClickOnSite provides tools to store tower infrastructure data such as energy parameters, battery life, alarms, fuel usage, and temperature of the tower site.
- Activities happening in and around the telecom tower can be recorded for any future requirement.
- Constant monitoring of infrastructures like energy consumption, battery charging, cooling system, and overall asset visibility is essential for the smooth functioning of cell towers.
- The main advantage of predictive maintenance is to act in a timely manner, which reduces downtime and increases infrastructure availability.
- Optimal use of the equipment throughout its life cycle.
Conclusion
Selecting a successful maintenance strategy requires a good knowledge of maintenance management principles and practices as well as knowledge of specific facility performance. There is no one correct formula for maintenance strategy selection and, more often than not, the selection process involves a mix of different maintenance strategies to suit the specific facility performance and conditions.High-value equipment-intensive utility industries that successfully adopt strategic maintenance management practices can reduce maintenance costs and improve productivity through superior planning and may become more responsive to market demands through improved flexibility and agility.
ClickOnSite can help create, manage and evaluate the maintenance strategy. It provides a multitude of services that will supercharge internal team, business, and maintenance operations.
References:
[1] European norm EN-13306 AFNOR. Maintenance Terminology. 2018.
[2] Kojchen K. and Monchy F. Maintenance: outils, méthodes et organisations efficientes.
Dunod, 2019.
[3] Ran Y., Zhou X., and Lin P. et al. A survey of predictive maintenance: Systems,
purposes, and approaches. IEEE Communication Surveys and Tutorials, 2019.
[4] R. Louhichi, Elaboration of an economic model for decision aid optimizing the
maintenance strategy of transport systems. Thèse Université de Technologie de Compiègne,
2021.
[5] Bouami D. Le grand livre de la maintenance. Afnor Éditions, 2019.
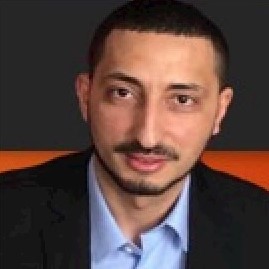
Mohamed Sallak
Ph.D. and Associate Professor/HRD at Heudiasyc Laboratory in Technology University of Compiègne (FRANCE)
Mohamed SALLAK
Ph.D. and Associate Professor/HRD
at Heudiasyc Laboratory
in Technology University
of Compiègne (FRANCE)
More information on his background,
research fields, projects, and other publications here.